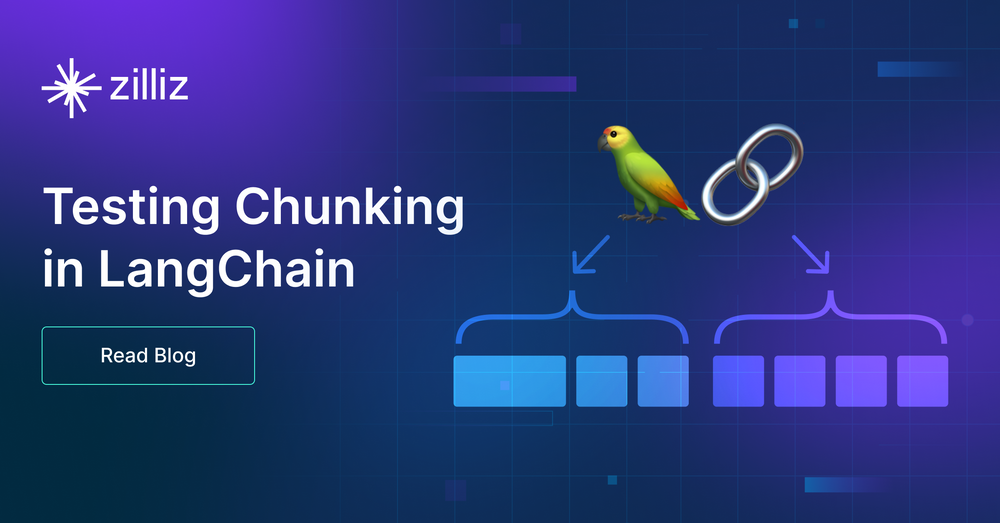
分块(Chunking)是构建检索增强型生成(RAG)应用程序中最具挑战性的问题。分块是指切分文本的过程,虽然听起来非常简单,但要处理的细节问题不少。根据文本内容的类型,需要采用不同的分块策略。
在本教程中,我们将针对同一个文本采用不同的分块策略,探索不同分块策略的效果。访问链接获取本文中涉及的代码。
01.LangChain 分块简介
LangChain 是一个 LLM 协调框架,内置了一些用于分块以及加载文档的工具。本次分块教程主要围绕设置分块参数,并最小限度地使用 LLM。简而言之,通过编写一个函数并设置其参数来加载文档并对文档进行分块,该函数打印结果为分块后的文本块。在下述实验中,我们会在这个函数中运行多个参数值。
LangChain 分块代码导入和设置
代码第一部分主要是导入和设置工具。下面代码有很多导入语句,os
和dotenv
都比较常用。它们仅用于环境变量。
接下来,我们深入讲解一下有关 LangChain 和 pymilvus 部分的代码。
首先是用于获取文档的三个导入:
NotionDirectoryLoader
用于加载含有 markdown/Notion 文档的目录。然后,MarkdownHeader 和 RecursiveCharacter 文本分割器会根据标题(标题分割器)或一组预先选定的字符分隔符(递归分割器)分割 markdown 文档中的文本。
接下来,是检索器导入。我们用 Milvus 、OpenAIEmbeddings 模型和 OpenAI 大语言模型(LLM)。SelfQueryRetriever 是 LangChain 原生检索器,允许向量数据库“查询自身”。
最后一个 LangChain 导入是AttributeInfo
,它将一个带有信息的属性传入 SelfQueryRetriever。
至于 pymilvus
导入,通常我只将这些导入在结束时用于清理数据库。
编写函数之前的最后一步是加载环境变量并声明一些常量。headers_to_split_on
变量列出了我们希望在 markdown 中分割的所有标题;path
用于帮助 LangChain 了解在哪里找到 Notion 文档。
import osfrom langchain.document_loaders import NotionDirectoryLoader
from langchain.text_splitter import MarkdownHeaderTextSplitter, RecursiveCharacterTextSplitter
from langchain.vectorstores import Milvus
from langchain.embeddings import OpenAIEmbeddings
from langchain.llms import OpenAI
from langchain.retrievers.self_query.base import SelfQueryRetriever
from langchain.chains.query_constructor.base import AttributeInfo
from pymilvus import connections, utility
from dotenv import load_dotenv
load_dotenv()
zilliz_uri = os.getenv("ZILLIZ_CLUSTER_01_URI")
zilliz_token = os.getenv("ZILLIZ_CLUSTER_01_TOKEN")
headers_to_split_on = [
("##", "Section"),
]
path='./notion_docs'
构建一个分块实验函数
构建分块实验函数是本教程中最关键的部分。如前所述,此函数需要一些参数用于档导入和分块。我们需要提供文档的路径、要分割的标题(分割器)、分块大小、分块重叠(chunk overlap)以及我们是否希望通过删除 Collection 来清理数据库。默认情况下,将该参数设置为 True,即删除 Collection 清理数据库。
注意,要尽可能少地创建和删除 Collection,从而避免不必要的开销。
函数第一部分通过 Notion 目录加载器(Notion Directory Loader)从路径加载文档,此处只抓取第一页的内容。
接下来,获取分割器。首先,使用 markdown 分割器根据上面传入的标题进行分割。然后,用递归分割器根据分块大小和 overlap 来分割。
分割完成后,使用环境变量、OpenAI embedding、分块工具以及 Collection名 称初始化一个 LangChain Milvus 实例。此外,我们还通过 AttributeInfo
对象创建了一个元数据字段列表,帮助 SelfQueryRetriever 了解文本块所属的“章节”。
完成所有上述设置后,获取 LLM 并将其传递给 SelfQueryRetriever。当我们针对文档提出问题时,检索器开始发挥作用。我还设置了函数从而了解其正在测试哪种分块策略。最后,可以按需删除 Collection。
def test_langchain_chunking(docs_path, splitters, chunk_size, chunk_overlap, drop_collection=True):
path=docs_path
loader = NotionDirectoryLoader(path)
docs = loader.load()
md_file=docs[0].page_content
# Let's create groups based on the section headers in our page
markdown_splitter = MarkdownHeaderTextSplitter(headers_to_split_on=splitters)
md_header_splits = markdown_splitter.split_text(md_file)
# Define our text splitter
text_splitter = RecursiveCharacterTextSplitter(chunk_size=chunk_size, chunk_overlap=chunk_overlap)
all_splits = text_splitter.split_documents(md_header_splits)
test_collection_name = f"EngineeringNotionDoc_{chunk_size}_{chunk_overlap}"
vectordb = Milvus.from_documents(documents=all_splits,
embedding=OpenAIEmbeddings(),
connection_args={"uri": zilliz_uri,
"token": zilliz_token},
collection_name=test_collection_name)
metadata_fields_info = [
AttributeInfo(
name="Section",
description="Part of the document that the text comes from",
type="string or list[string]"
),
]
document_content_description = "Major sections of the document"
llm = OpenAI(temperature=0)
retriever = SelfQueryRetriever.from_llm(llm, vectordb, document_content_description, metadata_fields_info, verbose=True)
res = retriever.get_relevant_documents("What makes a distinguished engineer?")
print(f"""Responses from chunking strategy:
{chunk_size}, {chunk_overlap}""")
for doc in res:
print(doc)
# this is just for rough cleanup, we can improve this# lots of user considerations to understand for real experimentation use cases thoughif drop_collection:
connections.connect(uri=zilliz_uri, token=zilliz_token)
utility.drop_collection(test_collection_name)
02.LangChain 分块实验和结果
接下来就是激动人心的时刻了!让我们来看看分块实验的结果。
测试 LangChain 分块
以下代码块展示了如何运行我们的实验函数。我添加了五个实验,这个教程测试的分块长度从 32 到 64、128、256、512 不等,分块 overlap 从 4 到 8、16、32、64 不等的分块策略。为了测试,我们遍历元组列表并调用上面写的函数。
chunking_tests = [(32, 4), (64, 8), (128, 16), (256, 32), (512, 64)]for test in chunking_tests:
test_langchain_chunking(path, headers_to_split_on, test[0], test[1])
以下为输出结果。接着让我们来仔细观察每一组实验的输出结果。我们使用的测试问题是“What makes a distinguished engineer?”
分块长度 32,重叠 4
显而易见,32 的长度太短了,这种分块策略完全无效。
分块长度 64,重叠 8
这种策略一开始效果也不理想,但最终也给出了问题的答案—— Werner Vogels,亚马逊(Amazon)首席技术官(CTO)。
分块长度 128,重叠 16
长度变为 128 时,答案出现了更多完整句,更少“工程师”类型的回答。这个策略的效果还不错,能够提取出 Werner Vogel 相关文本片段。但是这个策略的一个劣势是答案中会出现 \xa0
和 \n
这种特殊字符。也许我们分块长度过长了。
分块长度 256,重叠 32
虽然答案会返回相关内容,但这个分块长度过长。
分块长度 512,重叠 64
已知 256 的分块长度已经过长了。但是将长度设置为 512 时,会提取出整个 section 的内容。这时候就要思考:我们到底是想要结果中返回单独的一行文字,还是整个 section 内容?这就需要根据使用场景进行判断。
03.总结
本教程探索了 5 种不同分块策略的效果。选择分块策略时,我们要根据期望获得的返回结果来确定最合适的分块长度,后续我们将测试不同分块 overlap 的效果。敬请期待!