RAG开发中,如何用Milvus 2.5 BM25算法实现混合搜索
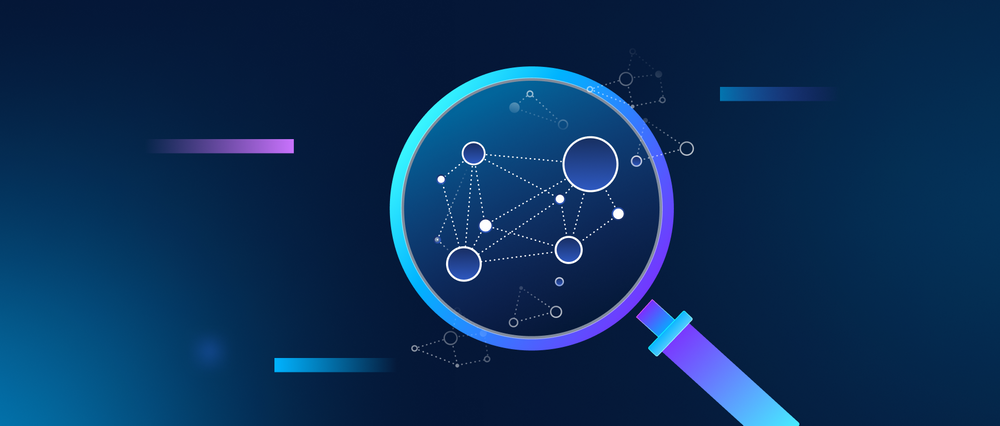
背景
混合搜索(Hybrid Search)作为RAG应用中Retrieve重要的一环,通常指的是将向量搜索与基于关键词的搜索(全文检索)相结合,并使用RRF算法合并、并重排两种不同检索的结果,最终来提高数据的召回率。全文检索与语义检索不是非此即彼的关系。我们需要同时兼顾语义理解和精确的关键字匹配。比如学术论文的写作中,用户不仅希望在搜索结果看到与搜索查询相关的概念,同时也希望保留查询中使用的原始信息返回搜索结果,比如基于一些特殊术语和名称。因此,许多搜索应用正在采用混合搜索方法,结合两种方法的优势,以平衡灵活的语义相关性和可预测的精确关键字匹配。
从 Milvus 2.4 版本开始,我们引入了多向量搜索和执行混合搜索(多向量搜索)的能力。混合搜索允许用户同时搜索跨多个向量列的内容。这个功能使得可以结合多模态搜索、混合稀疏和全文关键词搜索、密集向量搜索以及混合密集和全文搜索,提供多样且灵活的搜索功能,增强了我们的向量相似性搜索和数据分析。
Milvus BM25
在最新的Milvus 2.5里,我们带来了“全新”的全文检索能力
对于全文检索基于的 BM25 算法,我们采用的是 Sparse-BM25,基于 Sparse Vector 实现的 BM25 在存储效率、检索性能上都打开了更多的空间,同时也融合在了 Milvus 以向量为核心检索范式的产品理念里;
同时引入了原始文本插入和查询的能力,不需要用户手动将文本转成 Sparse Vector,这使得 Milvus 朝着非结构化数据处理的方向迈进了一步。
详情请参见 Milvus 2.5:全文检索上线,标量过滤提速,易用性再突破
Sparse-BM25 其原理类似 Elasticsearch 和其他全文搜索系统中常用的BM25算法,但针对稀疏向量设计,可以实现相同效果的全文搜索功能。
具有数据剪枝功能的高效检索算法:通过剪枝来丢弃搜索查询中的低值稀疏向量,向量数据库可以显著减小索引大小并以最小的质量损失达成最优的性能。
带来进一步的性能优化:将词频表示为稀疏向量而不是倒排索引,可以实现其他基于向量的优化。比如:用图索引替代暴力扫描,实现更有效的搜索;乘积量化(PQ)/标量量化(SQ),进一步减少内存占用。
详情请参见 Elasticsearch vs 向量数据库:寻找最佳混合检索方案
Milvus BM25 Hybrid Search
首先,准备数据和问题,数据来自Milvus 2.5 release notes,且通过llama-index的SentenceWindowNodeParser
对于数据进行分块处理。
!wget https://raw.githubusercontent.com/milvus-io/milvus-docs/v2.5.x/site/en/release_notes.md -O milvus_2_5.md
documents = SimpleDirectoryReader(
input_files=["./milvus_2_5.md"]
).load_data()
# Create the sentence window node parser
node_parser = SentenceWindowNodeParser.from_defaults(
window_size=3,
window_metadata_key="window",
original_text_metadata_key="original_text",
)
# Extract nodes from documents
nodes = node_parser.get_nodes_from_documents(documents)
# query question
query = "What are the key features in milvus 2.5?"
其次,创建collection的schema以及索引,其中原始文本数据存于text
列,而Sparse-BM25数据存于sparse_bm25
列,这里需要通过转换Function来实现
bm25_function = Function(
name="bm25",
function_type=FunctionType.BM25,
input_field_names=["text"],
output_field_names="sparse_bm25",
)
schema = MilvusClient.create_schema(
auto_id=False,
enable_dynamic_field=True,
)
# Add fields to schema
schema.add_field(field_name="id", datatype=DataType.INT64, is_primary=True)
schema.add_field(field_name="text", datatype=DataType.VARCHAR, max_length=512, enable_analyzer=True)
schema.add_field(field_name="sparse_bm25", datatype=DataType.SPARSE_FLOAT_VECTOR)
schema.add_field(field_name="dense", datatype=DataType.FLOAT_VECTOR, dim=dense_dim)
bm25_function = Function(
name="bm25",
function_type=FunctionType.BM25,
input_field_names=["text"],
output_field_names="sparse_bm25",
)
schema.add_function(bm25_function)
index_params = client.prepare_index_params()
# Add indexes
index_params.add_index(
field_name="dense",
index_name="dense_index",
index_type="IVF_FLAT",
metric_type="IP",
params={"nlist": 128},
)
index_params.add_index(
field_name="sparse_bm25",
index_name="sparse_bm25_index",
index_type="SPARSE_WAND",
metric_type="BM25"
)
# Create collection
client.create_collection(
collection_name=collection_name,
schema=schema,
index_params=index_params
)
然后,把数据进行Embedding之后,插入到Collection里,这里Embedding采用的是 OpenAI的 text-embedding-3-large
def gen_embedding(docs):
model_name = "text-embedding-3-large"
openai_ef = model.dense.OpenAIEmbeddingFunction(
model_name=model_name,
api_key=os.environ["OPENAI_API_KEY"]
)
return openai_ef.encode_documents(docs)
docs_embeddings = gen_embedding(docs)
query_embeddings = gen_embedding([query])
# Assemble data
data = [
{"id": idx, "dense": docs_embeddings[idx].data, "text": doc}
for idx, doc in enumerate(docs)
]
# Insert data
res = client.insert(
collection_name=collection_name,
data=data
)
最后,进行查询测试
4.1. 我们先测试下普通查询
search_params = {
"metric_type": "IP",
"params": {"nprobe": 10}
}
res = client.search(
collection_name=collection_name,
data=[query_embeddings[0]],
anns_field="dense",
limit=5,
search_params=search_params,
output_fields=["text"]
)
查询结果
TopK results:
0
0 Enhancements in cluster management, indexing, and data handling introduce new levels of flexibil...
1 With this release, Milvus integrates powerful new features like term-based search, clustering co...
2 Milvus 2.5 introduces a built-in Cluster Management WebUI, reducing system maintenance difficult...
3 \n\nv2.5.0-beta\n\nRelease date: November 26, 2024\n\n| Milvus version | Python SDK version | No...
4 \n\nRelease Notes\n\nFind out what’s new in Milvus!
从查询结果来看,最后一条召回内容与查询问题相关度不大。
4.2. 然后进行Hybrid Search。定义向量搜索和Sparse-BM25搜索
k=5 # get the top 5 docs related to the query
search_params_dense: { "metric_type": "IP", "params": {"nprobe": 10}}
request_dense = AnnSearchRequest([query_embeddings[0].data], "dense", search_params_dense, limit=k)
search_params_bm25 = {"metric_type": "BM25"}
request_bm25 = AnnSearchRequest([query], "sparse_bm25", search_params_bm25, limit=k)
reqs = [request_dense, request_bm25]
这里使用RRFRanker
来进行Hybrid Search
ranker = RRFRanker(100)
res = client.hybrid_search(
collection_name=collection_name,
reqs=reqs,
ranker=ranker,
limit=5,
output_fields=["text"]
)
for hits in res:
print("TopK results:")
for hit in hits:
print(hit)
查询结果:
TopK results:
0
0 \n\nv2.5.0-beta\n\nRelease date: November 26, 2024\n\n| Milvus version | Python SDK version | No...
1 Enhancements in cluster management, indexing, and data handling introduce new levels of flexibil...
2 This feature is disabled by default in Milvus 2.5 and will be officially available in version 3....
3 With this release, Milvus integrates powerful new features like term-based search, clustering co...
4 Powered by Tantivy, Milvus 2.5 has built-in analyzers and sparse vector extraction, extending th...
从结果来看,基于Sparse-BM25的Hybrid Search可以准确找到与查询相关的内容。相对于普通查询,召回的内容准确度更大。
总结:
本文讲述了Milvus 2.5中引入的Sparse-BM25基础原理,以及如何利用BM25算法实现RAG开发中的Hybrid Search(混合搜索)实践。通过引入Sparse-BM25算法,Milvus能够在稀疏向量上执行高效的全文检索,并与密集向量搜索相结合,提升检索的召回率和精确度。
参考文档:
https://zilliz.com/blog/hybrid-search-with-milvus
https://milvus.io/docs/multi-vector-search.md
代码可通过链接获取:https://pan.baidu.com/s/1eArbrvqmkYTJ-DS8eDkbJA?pwd=1234 提取码: 1234
技术干货
门槛一降再降,易用性大幅提升!Milvus 2.2.12 持续升级中
一句话总结 Milvus 2.2.12 :低门槛、高可用、强性能。
2023-7-27技术干货
LLM 快人一步的秘籍 —— Zilliz Cloud,热门功能详解来啦!
此次我们在进行版本更新的同时,也增加了多项新功能。其中,数据迁移(Migration from Milvus)、数据的备份和恢复(Backup and Restore)得到了很多用户的关注。本文将从操作和设计思路的层面出发,带你逐一拆解 Zilliz Cloud 的【热门功能】。
2023-4-10技术干货
当一个程序员决定穿上粉裤子
如何找到和你时尚风格相似的明星?AI + Milvus=?
2023-8-23